Made by Humans: The AI Condition
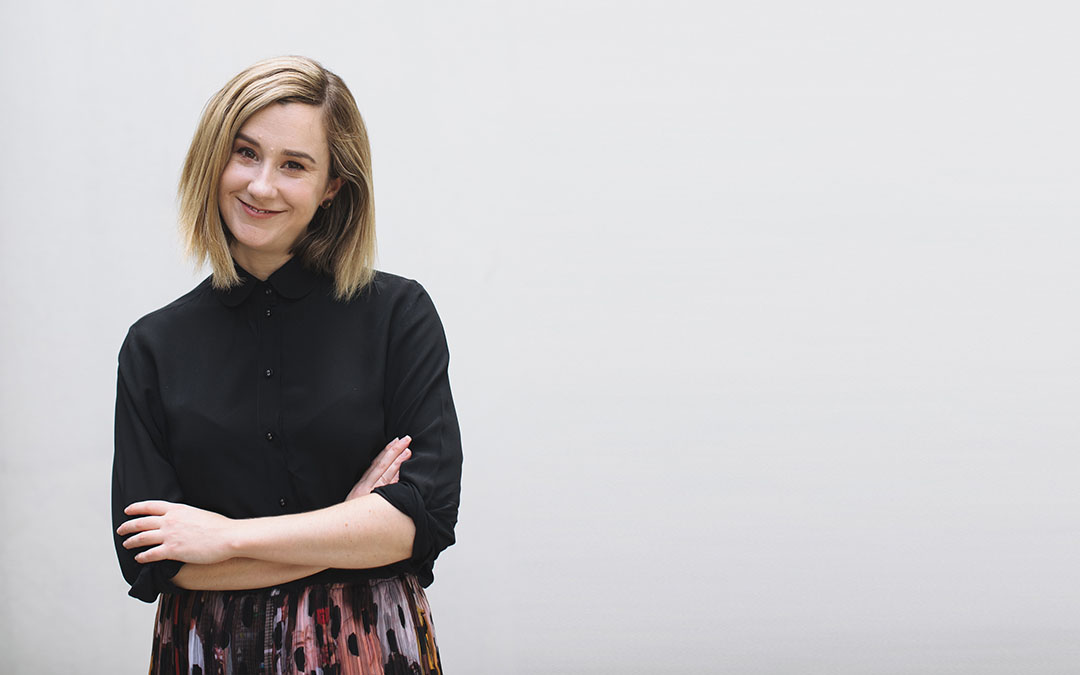
Ellen Broad is an independent data consultant and author. This article is an edited extract from Ellen’s book, Made by Humans: The AI Condition, available now through MUP. Ellen will speak on the relationship between humans and AI at Better Worlds in Sydney on 19 September 2018.
Rahimi was presenting as one of the recipients of NIPS’s Test of Time Award alongside UC Berkeley Professor Ben Recht. They wrote their paper about random kitchen sinks, a method for speeding up optimisation problems, over a decade earlier. If you watch Rahimi’s NIPS talk online, you will miss Recht, who was standing next to Rahimi on the stage in a T-shirt that read: ‘Corporate Conferences Still Suck’—an echo of Kurt Cobain on the cover of Rolling Stone magazine more than twenty years earlier with the tagline ‘Corporate Magazines Still Suck’ scrawled across his T-shirt in black marker.
Machine learning is often described as being a ‘black box’: precisely how it works and how decisions are made are impenetrable. What happens between practitioners inputting lots of data and getting their results can be unclear. This is not true of all machine learning models; some are more intelligible—that is, it is easier to trace through their decision-making process and understand them—than others. The problem is, the least intelligible methods tend to be more accurate. More intelligible methods, like linear regression, sometimes produce less accurate results.
Some machine learning practitioners talk about ‘black box’ machine learning with a kind of acceptance. This has the unsettling effect of making black box issues around some machine learning models seem innate, unchangeable—shortcomings to be tolerated in order to make progress. Rahimi argued that practitioners weren’t interested enough in trying to understand the black box. He called for more simple experiments and simple theorems, more focus on uncovering the reasons for puzzling bugs and strange machine learning phenomena. More rigour, basically—less alchemy.
Rahimi—and Recht, who co-wrote the acceptance talk— created a sensation across NIPS. Facebook’s Director of AI Research, Yann LeCun, came out the next day calling Rahimi’s alchemy metaphor “insulting” and “wrong”. LeCun was worried that describing machine learning as “alchemy”—dangerous and mysterious magic practised by unscientific people—could precipitate yet another AI winter: the cessation of funding and general support for AI-related research.
The argument between Rahimi and LeCun is an old one. Whether technological progress is measured in experimental breakthroughs, in the implementation of those breakthroughs in products, or in unpacking, understanding and theorising those breakthroughs, has long been debated. The relationship between risk-taking and responsibility has been examined by mathematicians, scientists and philosophers throughout history. Russian American mathematician and popular author Lillian Lieber, who counted Albert Einstein and Eric Temple Bell among her fans, wrote about the capacity of mathematics to “shed light on both the CAPABILITIES and the LIMITATIONS of the human mind”. Engineers who mistook licence (to take risk) for absolute freedom to do so, Lieber wrote, often resulted in “juvenile delinquency”.
Science needs breakthroughs and science needs caution. There are brilliant innovations that change our lives. Useless applications also get traction in the hype. And sometimes there are applications that are so imaginatively cruel as to leave us stunned, shaken by what the human mind is capable of dreaming up and what humans—as researchers, designers, funders and institutions—are willing to carry out.
The discovery of electricity, for example—to which AI is so frequently compared—took over two hundred years to move from novel discovery to practical, widespread application. It unlocked profoundly modernising forces like streetlights, safe forms of internal heating, home appliances, computers. It also unleashed the electric chair. Electric baths, shocks and massages were thought by the medical profession to be effective treatments for everything from blindness to rheumatism, from hysteria to headaches, for nearly a century. The utility—and harm—of electroconvulsive therapies is still debated in psychiatry today.
In his response to Rahimi’s alchemy metaphor at NIPS, LeCun argued that engineering artefacts had always preceded theoretical understanding, using examples like the steam engine and aeroplane. It’s also true that engineering breakthroughs that preceded theoretical understanding of those breakthroughs have occasionally been terrible failures. In the 1950s, the British Overseas Airways Corporation launched the world’s first commercial jet airliner, the de Havilland Comet, and looked poised to kick-start the modern jet age. But then three Comets broke up in mid-air within twelve months, killing everyone on board. Production of the British jet was halted. The investigations that followed transformed aviation safety, improving construction techniques, and resulting in the transition of design features like square windows on planes to round ones (corners concentrate stresses while curves distribute them). The history of the aviation industry is one of technical innovation, of devastating failure, and of lessons learned from failure. A complex ecosystem of laws, standards, best practices and institutions have grown up around the aviation industry along the way.
In describing practices in machine learning in 2017 as alchemy, Rahimi wasn’t calling for a stop to machine learning. He also wasn’t saying alchemy was bad: there were incredible, enduring discoveries alongside completely misplaced ones. He was asking for less complacency within the machine learning community in the face of its mysteries.
Machine learning is moving from experimental research to widespread application in ways that intimately affect people’s lives. Some of these applications are going to be robust, effective, rigorous. And some are going to be a waste of money. Some will turn out to be the equivalent of treating blindness with electric shocks. Some will cause harm.
LeCun was right to be concerned that a general perception of machine learning as alchemy—mysterious, dangerous, misdirected—could result in AI research losing funding again. But he didn’t dispute that aspects of machine learning aren’t currently understood by the people building the systems. It’s in the industry’s interests to change that. The machine learning industry will benefit greatly from investing as heavily in AI safety, fairness and transparency as it does in ‘new tricks’.

Stay in the loop
To receive updates about AgileAus and be subscribed to the mailing list, send us an email with your first name, last name and email address to signup@agileaustralia.com.au.
0 Comments