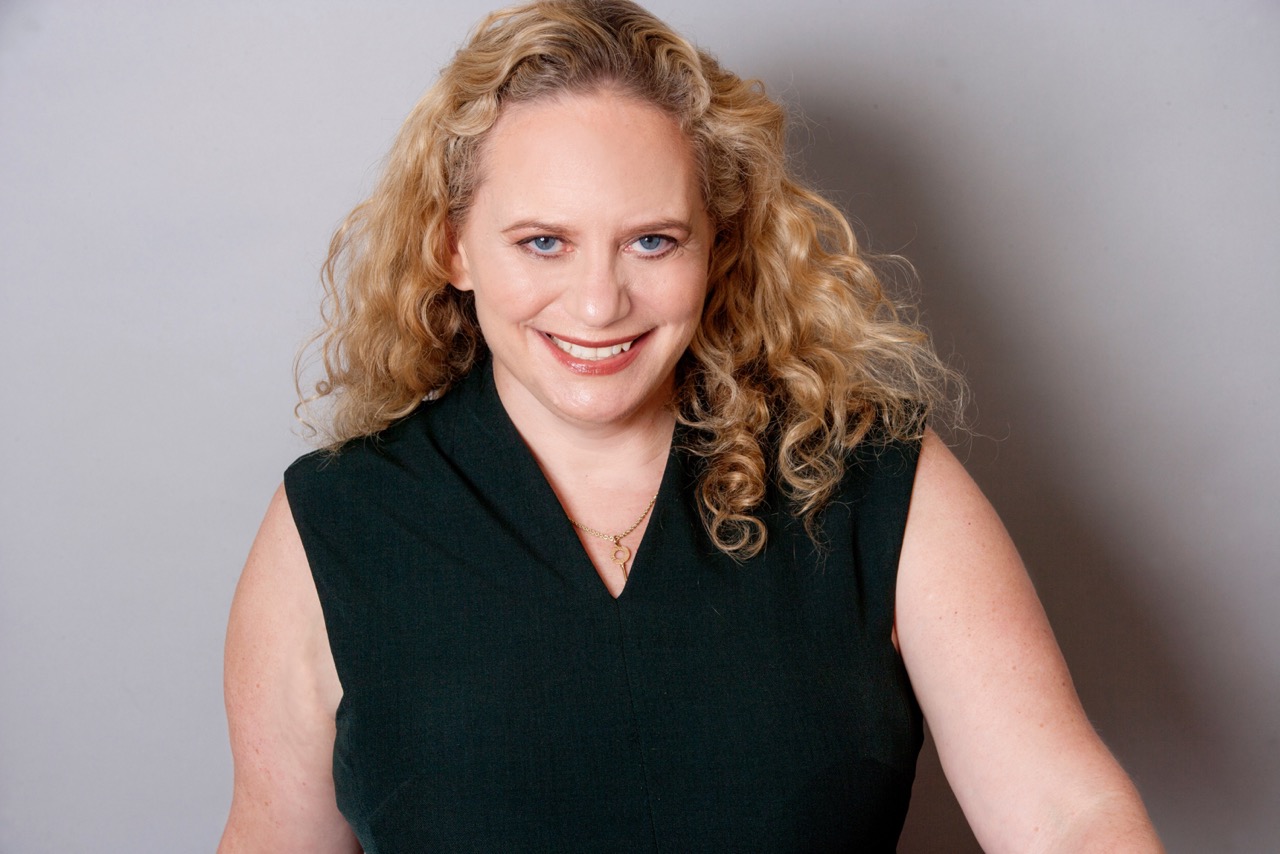
Agile Lessons from AI
Melinda Harrington reviews Made by Humans: The AI Condition by Ellen Broad (Melbourne University Press, 2018). Melinda is a Lead Consultant at Elabor8. She is an Agile coach, a speaker, a writer, a blogger, and a passionate believer that we can always improve the way we work.
Those of us who strive to be Agile are likely to find relevance in Ellen Broad’s Made by Humans: The AI Condition. Primarily, it’s because many of us work in technology and may use Artificial Intelligence now or in the future. Secondly, because whether we are thinking about AI or not, many themes in Broad’s book are those we are already grappling with in the Agile domain. These challenges are extensions of those to which we are accustomed. As ‘Agilists’, we are uniquely prepared to understand the human side of technology. We have a responsibility to consider the impact of our choices and actions.
When thinking of humanity, a good place to start is with ourselves. Broad opens this book with her own story. Her journey is not superfluous; it is integral. Although AI is another level of computer power, it continues to be “made by humans”. It’s important to understand who those humans are and how they select and interpret data.
The obvious way to frame the likelihood of data being incorrect is to query the data that is held about us. Motivated by this book, I downloaded the data that Facebook holds about me. I find it humorous that Al Smith Chrysler Dodge Jeep Ram Inc. in Bowling Green, Ohio uploaded a contact list with my information to Facebook. It’s funny because the likelihood of me buying a car from them is zero.
It becomes less humorous when incorrect information is being used to draw conclusions about me. Given the number of car dealerships weirdly associated with my profile, it may be erroneously assumed that I am a rev-head. What happens if banks issuing mortgages decide that rev-heads aren’t a good risk? What if I was denied a loan based on this inaccurate information? This is a theoretical assumption but similar real-life examples abound.
Machine learning is trained on data. Where that data comes from, what (or who) is included and excluded is selected by human beings. Broad’s observation: “What worries me isn’t so much that my data is ‘out there’, but that the industry being built off this kind of information has absolutely no idea how to use it properly.” (49)
I am wary of AI. It seems to be the latest buzz word, it is potentially quite dangerous and sometimes it is just too hard to comprehend. Reading this book has given me a better understanding. When AI seems too futuristic, I am reminded of the technology we now take for granted. The idea of AI might make me queasy in the abstract but speech recognition and spam filtering don’t worry me at all. What is difficult to comprehend now will soon become normal. Nevertheless, designing complex systems can be dangerous for the end user. “If you don’t understand how a system works, how can you be sure you’ve built the system safely?” (86) Broad asks.
Explaining complex concepts in a way that non-technical people can understand is a skill that is practiced in the Agile community. With our emphasis on customer collaboration and the adoption of user stories, we have become accustomed to describing our work to non-technical people. As challenging as that is with traditional programming, it might actually be too difficult for the people designing machine learning systems. “There is a difference between what can be explained, and what can be explained in a way so as to be understood.” (85)
Broad cautions: “Intelligibility of AI cannot just be left to organisations and practitioners designing AI. Mechanisms for improving intelligibility should envisage the growth of a range of intermediaries to help people understand the impact of automated systems as well.” (85) In this domain, accuracy and intelligibility both need to be considered. For example, if the conclusions reached through machine learning had not been questioned by an individual in Broad’s example of the data-set Chest X-Ray (10), people could have been incorrectly diagnosed.
With transparency as a Kanban value and openness as a Scrum value, the concept is one we are familiar with. Broad adds nuance to these words that was new to me. In this context, ‘transparency’ is about making information available. ‘Openness’ adds the opportunity for those you share information with to have input into changing it. (103)
It is vital that we don’t hide behind the machines and lose sight of the impact of our work on people. In the Agile world, individuals and interactions are in the forefront. As we step further into the world of AI, we need to keep that focus. Sadly, there are many examples of experiments where Artificial Intelligence has had a detrimental effect on the lives of other human beings.
Empathy is a recurring theme in Made by Humans. There is no doubt this is a human-centered book with an emphasis on under-represented, vulnerable groups. Some may assume that computers are objective. However, they are as subjective as the people who program them. If we are not careful, computers may be more likely to amplify the subjective. As Broad explains, “The machine might end up reproducing bias more frequently than human decision makers would.” (31)
Many themes in Made by Humans have clear links to commonly discussed Agile concepts. However, Broad devotes a large section of her book to the importance of government regulation. This is a relevant lesson for all of us that we may not spend as much time considering as we should. Do we see regulation as an annoyance or something to embrace? Regulations do not just constrain us, they protect us. We should ensure that our governments understand and regulate the technologies that they utilise. What happens if people can’t challenge decisions that are made about them with information that is not true? “Should system designers be held accountable for statements about the accuracy of their decision-making systems that aren’t true?” (146). These questions haven’t been answered.
Mistakes made in this arena have real world consequences that are amplified by the sheer volume of data and people involved. Artificial Intelligence has great promise. However, as Broad makes clear: “Science needs breakthroughs and science needs caution.” (79) Let’s ensure we have both. People need to continue to be our priority while we explore the new possibilities that this technology brings.

Stay in the loop
To receive updates about AgileAus and be subscribed to the mailing list, send us an email with your first name, last name and email address to signup@agileaustralia.com.au.
0 Comments